Enabling the full potential of automotive 3D ToF imaging
By Gualtiero Bagnuoli, Melexis
Time-of-flight (ToF) has been considered for a while a niche and exotic technology within the automotive industry. Lack of automotive proven sensors and more in general a not yet mature ecosystem, resulting in high costs, were preventing from wider adoption of this technology.
Another restricting factor was the relatively low resolution of the ToF sensor itself, resulting in a limited set of use cases due to either a narrow field-of-view (FoV) or not enough spatial resolution with a wider field-of-view.
ToF technology has gained a new momentum because of additional active safety standards (e.g. NCAP) and features required by level 4 and 5 autonomous vehicles, going beyond in-cabin use. ToF technology is now evaluated for exterior use cases like short range cocooning because it combines high resolution with accurate depth information at short range, complementing long range system such as camera and radar.
It is clear that the variety of use cases implies the availability of a sensor with specific characteristics to enable the industry to choose the most appropriate solution and optimize performance/cost ratio.
The uniqueness of ToF technology is the capability to combine an ambient light insensitive image and high resolution distance measurement. Today 2D image sensing and radar have been widely adopted by the automotive industry.
For what concerns distance measurement, radar technology can be very powerful and detects very small movements but with limitation in terms of spatial resolution. ToF technology provides a medium resolution image along with distance, which could be considered as a combination of 2D image sensors and radar.
Melexis started to invest in this technology because depending on the requirements, ToF can either replace the two above mentioned technologies or be complementary to them to provide high reliability. ToF based systems are gaining significant interest as more use cases are supported by a single system.
When the overall system cost of a 2D+NIR system and a ToF 3D system are compared, a significant part is the processor. 2D+NIR systems will typically have a larger resolution (one million pixels, evolving to two million pixels and even four million pixels), which means the 2D+NIR processor will need to process more pixels, so a bigger and thus more expensive processor is needed for 2D.
While with ToF, depth information is already available, 2D-based systems need extra processing for estimating depth information, so once more 2D systems will need more processing for similar functions. Even with more processing, 2D systems will suffer from significantly lower depth accuracy compared to ToF. When high depth accuracy is needed, ToF is clearly the technology of choice.
Earlier the emphasis was put on the availability of automotive proven ToF sensors, today the industry needs a complete portfolio as presented by Melexis. Let’s take two typical use cases: gesture recognition and in-cabin monitoring.
Gesture recognition
The first generation of gesture recognition systems is usually limited to a restricted interaction area, usually around the central console. The field-of-view is relatively narrow, the distance range is limited but depth precision is already good. A QVGA resolution (320 x 240 px) was sufficient and cost-effective LED illumination could deliver the required performance.
With the same resolution, it is possible to use a wider field-of-view in order to cover both front seats. The tracking of arm, head and in general larger objects will still be possible but not the tracking of fingers.
For the next generation gesture recognition systems, the goal is to continue to do finger detection in a wider zone. For this, a ToF sensor with higher resolution, such as VGA, will be needed for covering a wide field-of-view with enough spatial resolution required to properly detect and track fingers as well as for the seats at the back of the vehicle.
In-cabin monitoring
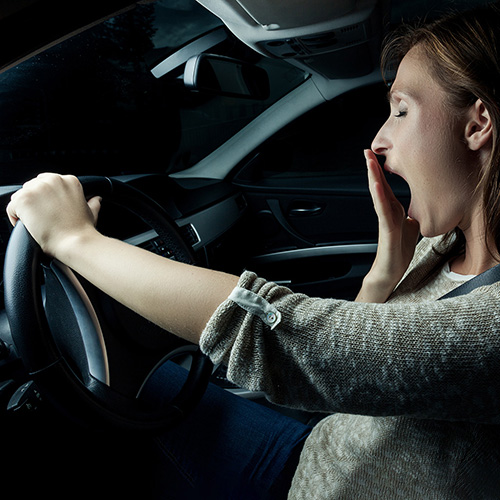
Driver sensing for NCAP and L3, L4 autonomous drive handover
- Body pose
- Hands-on-wheel
- Head pose
- Eyes open-close
- Eye gaze
- Fatigue
- Cognitive load
- Hand position to detect smartphone, drinks, etc.
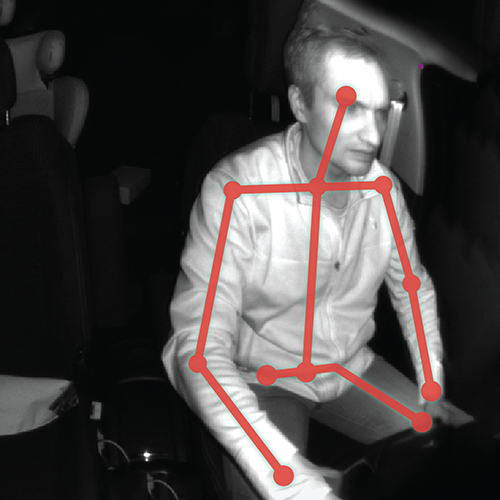
Personalization with body, head and face monitoring
- Driver and passenger with one sensor
- Body monitoring for seat and mirror adjustment
- Head and Face monitoring for people identification
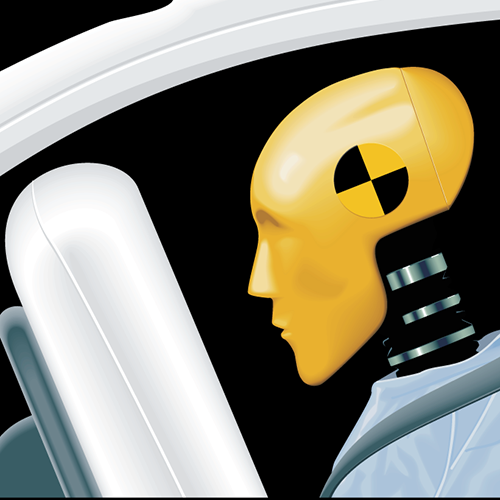
Active safety systems for NCAP and legal requirements
- Driver, passenger, child classification
- Smart airbag deployment
- Seatbelt detection
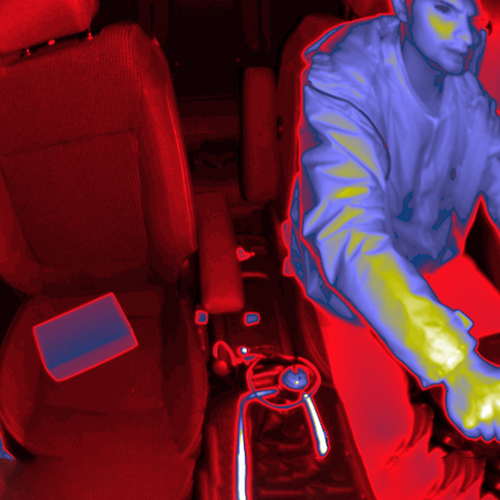
Object detection
- Objects left behind
- Parcel classification
People counting and the position of people in the vehicle can be carried out with a QVGA sensor (320 x 240 px).
When it comes to collecting detailed information of the driver’s biomechanical and cognitive state, higher resolution and depth precision become mandatory.
Through employment of 3D imaging data, the driver’s body pose, their head position and hand positioning can all be accurately ascertained. It can be confirmed, for example, that the driver’s attention is on the road and their hands are placed on the steering wheel, which is called “hands-on-wheel”.
The 3D information can be used to estimate the biomechanical reaction time of the driver to re-engage and to compare with the event horizon that the vehicle has calculated to gauge the safety margin. If the driver is not adequately engaged to react quickly enough, the ADAS is aware that should a potentially dangerous situation arise, it may be required to step in.
The use case described requires both higher and depth precision. Whenever high depth precision is required, cost-effective LEDs have to be replaced by VCSELs, which can operate at modulation frequency well beyond the typical maximum operating frequency of LEDs (up to 100 MHz for VCSELs).
Time-of-flight offering for automotive industry
Melexis has been pioneering in optical time-of-flight technology applied to the automobile industry.
Melexis’ automotive ToF portfolio is today the most complete one with more products coming up soon, letting customers to select the optimal sensor for their system in terms of precision, resolution and integration level.